Aligning Data Protection Principles with Emerging Tech
- CUHRLS
- Mar 14, 2023
- 5 min read
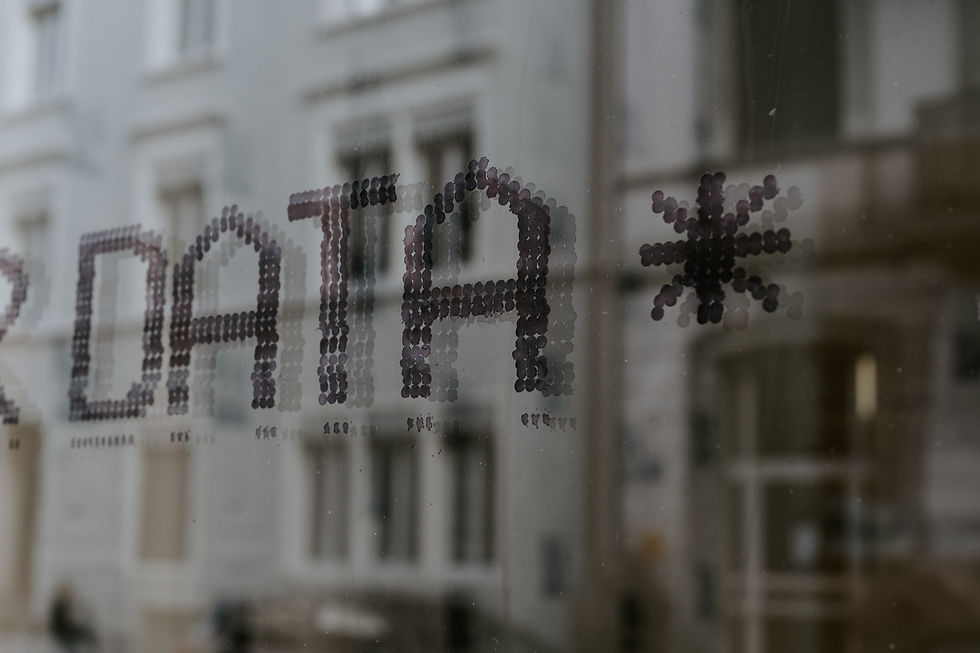
It is established that in a digitised world such as ours, data rights are human rights. With this pretext, we write this article to caution against the adoption of Artificial Intelligence ('AI') and its seemingly inevitable integration into our daily lives. Chat GPT recently became the fastest-growing consumer application in history, amassing 100 million+ active users in under two months, a feat that prominent social media sites such as TikTok and Instagram took 2.5 months and 2.5 years, respectively, to attain. At present, the chatbot is being used to automate tedious professional tasks such as writing emails and summarising and paraphrasing documents, amongst others. However, generative AI does more- it creates new data, including images, videos, music, simulations and codes. It has garnered attention for passing advanced exams, winning art competitions, explaining complex concepts, and many more potential applications that are actively being explored.
While the benefits associated with the technology cannot be negated, there are multiple concerns about its impact on the human rights of its users, including the right to privacy. Large Langauge Models ('LLMs') are trained on massive amounts of data and rely on self-supervised learning to generate outputs. In many cases, the datasets used to train these models contain personal and sensitive data often collected without adequate consent. Countries are increasingly taking steps to regulate the proliferation of AI to protect and enforce citizens' privacy rights, including data protection.
In this article, we primarily deal with the AI model's ability to allow for the exercise of data subject rights, particularly the right to be forgotten and the implementation of privacy principles, namely data minimisation and purpose limitation. The reasons behind choosing these two principles, in particular, are grounded in the fact that most commercially available AI consumer products are data intensive and often need a continual data flow to improve and provide effective solutions. In light of the same, multiple jurisdictions, such as the United Kingdom, the European Union, and the United States of America, have moved towards closely regulating the space due to its use of personal data and privacy implications. The Federal Trade Commission ('FTC') in early 2021 also forced a company to delete a collection of their data as they included personally identifiable data beyond the scope of their consented purpose. Thus, as we move towards a new frontier of AI integration into our lives, it is beneficial for all stakeholders if we tread with caution and enable a privacy-centric deployment.
AI and the Right to be Forgotten
The problem arises with exercising our data subject rights against artificial intelligence tools. Generative AI chatbots such as Chat GPT can retain individuals' personal information, whether inputted by the user (inputting your name and other personal information) or collected by the system (location, make of device and device details such as IP address etc.). While inputting personal information may enable personalised results, the primary contention arises with exercising the right to be forgotten or the right to correct and erase personal information. Technically, it has been an industry truth thatdata never leaves the system, even when deleted in most machine learning models. It merely gets delinked and pushed into the 'garbage offset' unit. The judicial complexity surrounding data deletion is often addressed. However, data deletion requisitions also have technical obstacles to pass. For instance, in the status quo, machine learning-based AI models are built in a manner that doesn't allow them to forget specific data points. Atop this, such models fall apart when numerous data deletion requests are sent quickly, further complicating any parlances with data subject rights being exercised effectively.
Since this problem of forgetting is not a new problem, multiple researchers are working to solve it. Proposed solutions revolve around implementing gap layers that quarantine data for a set time between training data and the learning algorithm to allow for a window where users can request deletion. Other solutions include allowing users to delete data points with a level of granularity while maintaining system integrity (i.e. the algorithm remains unaffected despite data deletion) or even a source-level segregation model that showed promise but was quickly proven to be susceptible to falter if data deletion requests happened to come in a specified sequence either by chance or through the actions of a malicious actor. However, 'Knowledge Unlearning', a data deletion model that sequentially deletes data instead of removing it all at once from the algorithm, has shown promise in enabling data deletion without significant impacts on the LM's ability to function. While the model shows promise, it needs to be assessed across a multitude of factors before we claim it to be the way forward.
How AI and Data Minimisation are Diametric
LLMs and the principles of data minimisation and purpose limitation stand at diametric ends. This is because the functionality of LLMs stands contingent on having vast volumes of data. Similarly, the principle of purpose limitation can also be argued to be in direct conflict with big data practices. To add further complication into the mix, how these models reach their conclusions is often unknown even by their creators, thereby making purpose limitation at the outset a near impossibility.
The arrival of generative AI fundamentally challenges how we perceive our data rights and thus warrants intervention from the state and industry alike. Solutions such as explainable AI and algorithmic accountability are being explored to increase the transparency of AI models. Human-centred approaches have also emerged in recent times, including the participatory machine learning approach, which focuses on involving a wide variety of stakeholders in the early development process to shape the goals and designs of the system. It is thus in the best interest of all stakeholders that we intervene at this stage and ensure that subject data rights aren't sidelined. Any AI-based technology rollout, therefore, needs to factor in these key data subject rights and how to enable redressal mechanisms in this time frame where adoption is still in its nascent stages. Any delay in implementing safeguards that enable data subject rights may eventually compound to a degree where either data subject rights are diluted, or entire AI models are re-trained.
Authors:
Garima Saxena: Garima Saxena is a Research Associate at The Dialogue, New Delhi. She pursued her undergraduate degree from Rajiv Gandhi National University of Law, Punjab, India. Her prime interest lies in how our society interacts with technology and its impact on individuals. She actively advocates for privacy and digital freedom through her work.
Bhavya Birla: Bhavya Birla is a Research Associate at The Dialogue, New Delhi. He pursued his undergraduate degree in law from Symbiosis Law School, Noida, India. His areas of interest cover informational privacy, privacy regulation, data flows, encryption etc. He is a proponent of digital privacy, freedom, and safety for all.
Comments